Starting with an initially simple Convolutional Neural Network (CNN), the precision and efficiency of a model can usually be increased step by step by arbitrarily scaling network dimensions such as width, depth, and resolution.
Increasing the number of levels used or using higher resolution images to train the models is usually associated with a high manual effort. Researchers at the Google Brain AI team now use EfficientNet to present a new scaling approach based on a fixed set of scaling coefficients and advances in AutoML.
Optimization across all network dimensions
Behind the EfficientNets hides a number of new models, which according to Google promise high precision with optimized efficiency (smaller and faster). Based on the AutoML MNAS Framework newly developed model EfficientNet-B0, which uses the architecture of Mobile Inverted Bottleneck Convolution (MBConv) – comparable to MobileNetV2 and MnasNet. The simple structure of this network should create the conditions for generalized scaling according to the new approach.
Instead of optimizing individual network dimensions independently of each other, Google researchers are now looking for a balanced scaling process across all network dimensions. The optimization starts with a grid search to determine the dependencies between the different dimensions with regard to parameters such as a doubling of the FLOPS. Then, the scaling coefficients thus obtained are applied to the network dimensions to scale a base network to the desired target model size or budget.
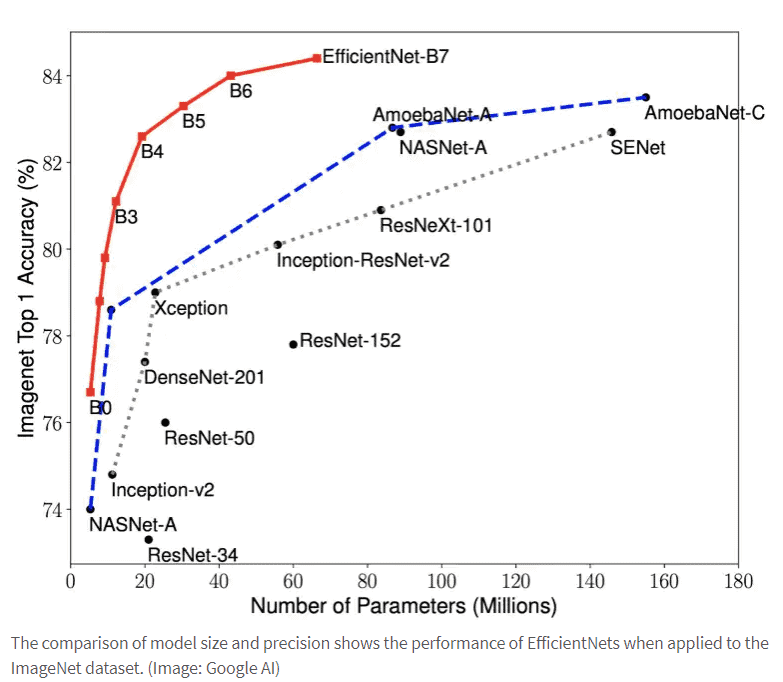
A detailed description of the new scaling approach behind the EfficientNet can be found in the Google AI blog post and in the ICML 2019 paper “EfficientNet: Rethinking Model Scaling for Convolutional Neural Networks”. Google provides the source code, including the TPU training scripts, as open source on the GitHub project page.